Supported by |
|
|
|
Additional cooperation from |
|
|
Sixth International Workshop on Symbolic-Neural Learning (SNL-2022)
July 8-9, 2022 Venue: Toyota Technological Institute, Nagoya
Notice: The order of speakers on July 8th has been changed due to unavoidable circumstances.
Keynote Talks:
- July 8 (Friday), JST 13:10-14:10
Katsushi Ikeuchi (Microsoft)
"Learning-from-observation: modeling human common-sense"
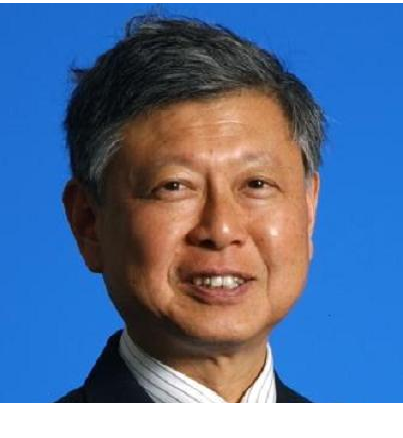
Abstract:
Our group has been developing Learning-from-observation systems (LfO), in which robots learn their actions by observing human demonstrations. LfO sounds similar more common Learning-from-Demonstration and Imitation learning, which mainly learn human hand/body trajectories from observation; however, with these bottom-up approaches, it is difficult for the robots to gain high-level knowledge of those actions and, as the results, those systems are susceptible to observation/demonstration errors and requires numerous demonstrations to achieve satisfactory results. Instead, we are working on a top-down approach, Learning-from-Observation, to organizes observation results into Minsky's frame like representations, task-models, derived from representing human common-sense. The system first understands what-to-do from observation and retrieves corresponding one task model and then, fills in the details of the task model, how-to-do, using various CNN-based observation modules. Having such high-level understandings of actions needs only a small number of observations by guiding the system where to look and facilitates error recovery of observations. It is also true that such high-level understandings can be used to design reward functions to train the robot execution modules by reinforcement learning. This presentation will focus on three classes of human common-sense used in our system and how to design the system using the common-sense as well as how to train the robot execution modules from the common-sense. (Ikeuchi IJCV2018, Ikeuchi arXiv:2103.02201, Saito arXiv2203.00733)
Bio:
Dr. Ikeuchi joined Microsoft in 2015, after working at MIT-AI, AIST-Japan, CMU-RI and U. Tokyo, His research interest spans computer vision, robotics, and ITS. He was EIC of IJCV and IJITS as well as Encyclopedia of Computer Vision. He general-program-chaired many international conferences including IROS95, CVPR96, ICCV03, ITSW07, ICRA09, ICPR12 and ICCV17. He received several awards, including IEEE-PAMI Distinguished Researcher Award, Okawa award, Funai award, IEICE outstanding achievements and contributions award as well as Medal of Honor with Purple ribbon from the Emperor of Japan. He is a fellow of IEEE, IAPR, IEICE, IPSJ and RSJ. He received a Ph.D degree in Information Engineering from the University of Tokyo and a Bachelor degree in Mechanical Engineering from Kyoto University. (http://www.cvl.iis.u-tokyo.ac.jp/~ki)
- July 8 (Friday), JST 14:10-15:10
Dan Roth (University of Pennsylvania/AWS AI Labs)
"On Reasoning, Planning, and Incidental Supervision"
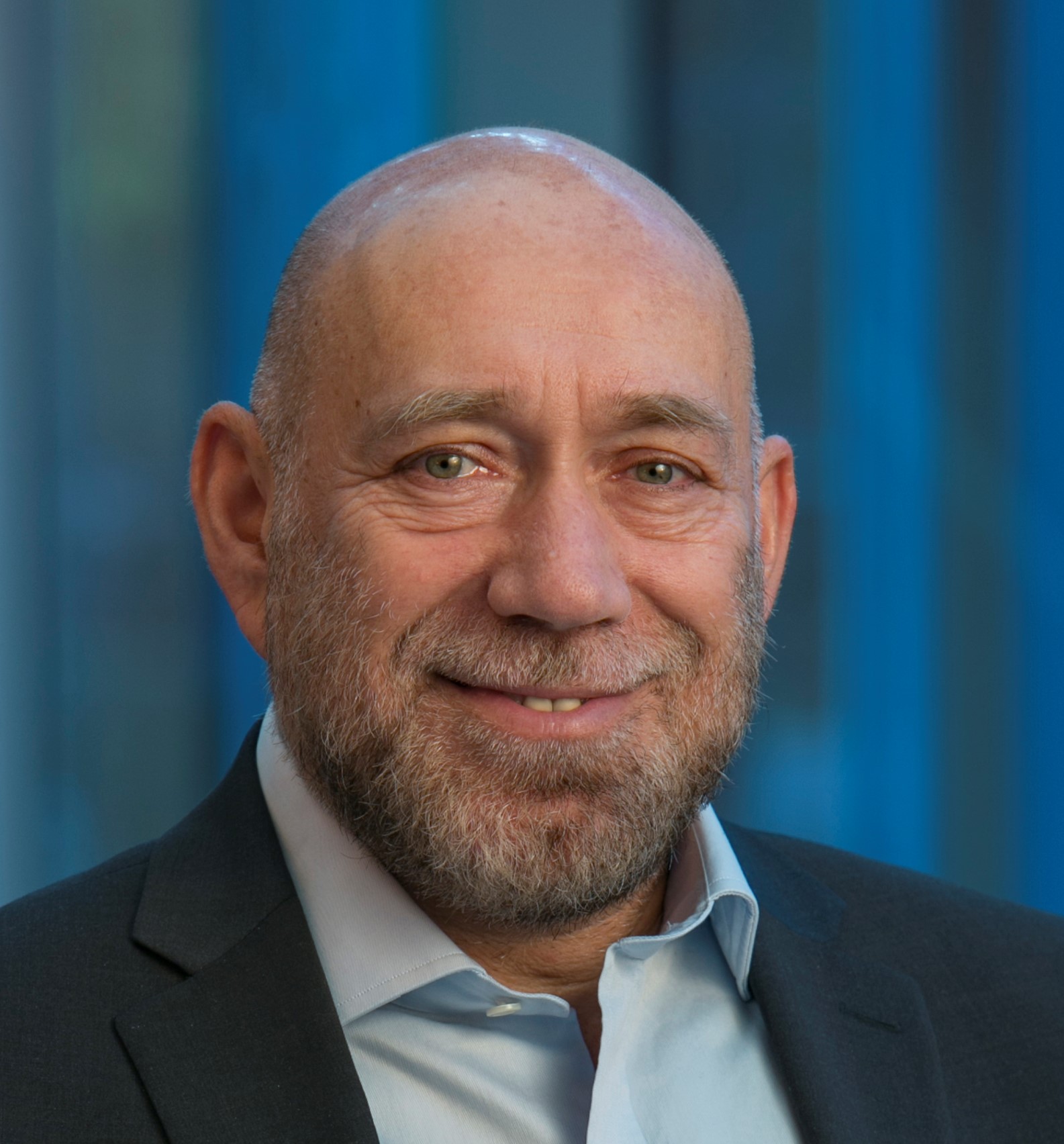
Abstract:
I will discuss some of the challenges underlying reasoning, how we should think about it in the context of natural language understanding, and how should we train for it. Reasoning ought to support natural language understanding decisions that depend on multiple, interdependent, models; it cannot be accomplished by "evaluating" a single model nor can we train directly to accomplish it. At the heart of it is a planning process that determines what modules are relevant and what knowledge needs to be accessed in order to support the decision. I will exemplify the needs and challenges using the domain of Reasoning about Time and Space, as expressed in natural language.
Bio:
Dan Roth is the Eduardo D. Glandt Distinguished Professor at the Department of
Computer and Information Science, University of Pennsylvania, lead of NLP Science
at Amazon AWS AI, and a Fellow of the AAAS, the ACM, AAAI, and the ACL.
In 2017 Roth was awarded the John McCarthy Award, the highest award the AI
community gives to mid-career AI researchers. Roth was recognized "for major conceptual
and theoretical advances in the modeling of natural language understanding,
machine learning, and reasoning."
Roth has published broadly in machine learning, natural language processing,
knowledge representation and reasoning, and learning theory, and has developed
advanced machine learning based tools for natural language applications that are
being used widely. Roth was the Editor-in-Chief of the Journal of Artificial
Intelligence Research (JAIR) and a program chair of AAAI, ACL, and CoNLL.
Roth has been involved in several startups; most recently he was a co-founder
and chief scientist of NexLP, a startup that leverages the latest advances in
Natural Language Processing (NLP), Cognitive Analytics, and Machine Learning
in the legal and compliance domains. NexLP was acquired by Reveal in 2020.
Prof. Roth received his B.A Summa cum laude in Mathematics from the Technion,
Israel, and his Ph.D. in Computer Science from Harvard University in 1995.
- July 9 (Saturday), JST 10:00-11:00
Sebastian Riedel (Facebook AI Reearch/UCL)
"Generative Retrieval"
![]()
Abstract:
Symbolic computation (e.g., in a Prolog interpreter) requires various ingredients. There need to be forms of sequence manipulation, a workspace to perform such manipulations, a mechanism to pursue several hypotheses (e.g. backtracking) and a way to retrieve relevant information such as rules from a knowledge base. In this talk I will argue that modern language models provide most of these ingredients but struggle with retrieval. I will then discuss our work on using generative language models for retrieval. Beyond being very effective in its own right, with state-of-the-art results across many diverse retrieval tasks, this approach opens a pathway towards language models as fully equiped symbolic computation engines.
Bio:
- July 9 (Saturday), JST 14:30-15:30
Eduard Hovy (University of Melbourne/CMU)
"The relationship between Symbolic and Neural NLP"
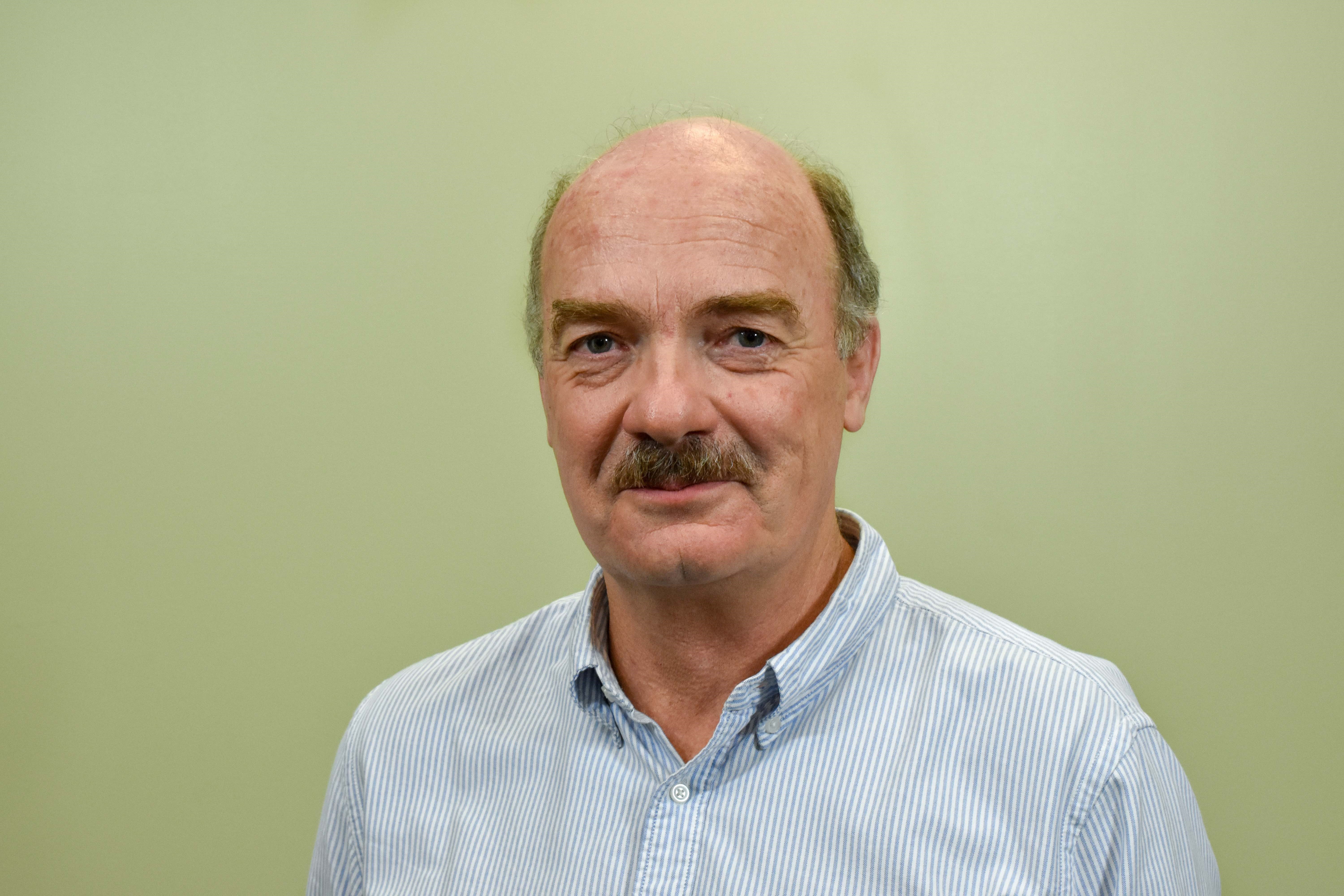
Abstract:
The relationship between neural and symbolic computing / machine learning has
become a very rich topic. Where some researchers feel strongly that more DNN (deep
neural network) engineering is enough to solve most of the problems of NLP, others
feel equally strongly that DNNs and large Language Models will never succeed without
some additional (usually symbolic) assistance. Yet the former cannot actually
demonstrate why or how and the latter cannot really prove or strongly justify their
beliefs. Both sides continue to explore: the former with new architectures,
encodings, and training methods, and the latter with symbolic-neural combinations of
various kinds. I propose we consider what canNOT be done, ask why, and try to reason
from basic principles. Should this approach succeed one can potentially save a lot
of investigation and rhetoric. In this talk I describe several methods one can
employ to determine what can and cannot be done, and use this to suggest a methodology
to guide future research in this fascinating interplay between symbolic and neural
language processing.
Bio:
Eduard Hovy is the Executive Director of Melbourne Connect (a research transfer centre
at the University of Melbourne), a professor at the university's School of Computing and
Information Systems, a research professor at the Language Technologies Institute in the
School of Computer Science at Carnegie Mellon University, and an adjunct professor in
CMU's Machine Learning Department. In 2020-21 he served as Program Manager in DARPA's
Information Innovation Office (I2O), where he managed programs in Natural Language
Technology and Data Analytics. Dr. Hovy completed a Ph.D. in Computer Science (Artificial
Intelligence) at Yale University in 1987 and was awarded honorary doctorates by the National
Distance Education University (UNED) in Madrid in 2013 and the University of Antwerp in 2015.
He is one of the initial 17 Fellows of the Association for Computational Linguistics (ACL)
and is also a Fellow of the Association for the Advancement of Artificial Intelligence (AAAI).
Dr. Hovy's research focuses on computational semantics of human language and addresses various
areas in Natural Language Processing and Data Analytics, including in-depth machine reading
of text, information extraction, automated text summarization, question answering, the
semi-automated construction of large lexicons and ontologies, and machine translation.
In early 2022 his Google h-index was 95, with over 54,000 citations. Dr. Hovy is the author
or co-editor of eight books and around 400 technical articles and is a popular invited speaker.
From 2003 to 2015 he was co-Director of Research for the Department of Homeland Security's
Center of Excellence for Command, Control, and Interoperability Data Analytics, a distributed
cooperation of 17 universities. In 2001 Dr. Hovy served as President of the international
Association of Computational Linguistics (ACL), in 2001-03 as President of the International
Association of Machine Translation (IAMT), and in 2010-11 as President of the Digital Government
Society (DGS). Dr. Hovy regularly co-teaches Ph.D.-level courses and has served on Advisory
and Review Boards for both research institutes and funding organizations in Germany, Italy,
Netherlands, Ireland, Singapore, and the USA.
Invited Talks:
- July 8 (Friday), JST 15:30-15:55
Saeed Seddighin (Toyota Technological Institute at Chicago)
"Playing the Election Game: Solving Blotto and Beyond"
Abstract:
The competition between the Republican and the Democrat nominees in
the U.S presidential election is known as Colonel Blotto in game
theory. In the classical Colonel Blotto game -- introduced by Borel in
1921 -- two colonels simultaneously distribute their troops across
multiple battlefields. The outcome of each battlefield is determined
by a winner-take-all rule, independently of other battlefields. In the
original formulation, the goal of each colonel is to win as many
battlefields as possible. The Colonel Blotto game and its extensions
have been used in a wide range of applications from political
campaigns (exemplified by the U.S presidential election) to marketing
campaigns, from (innovative) technology competitions, to sports
competitions. For almost a century, there have been persistent efforts
for finding the optimal strategies of the Colonel Blotto game,
however, it was left unanswered whether the optimal strategies are
polynomially tractable. In this talk, I will present several
algorithms for solving Blotto games in polynomial time and I will
explain their applications in practice.
Bio:
Saeed Seddighin is a Research Assistant Professor at TTIC. He spent 7
months as a postdoc at Harvard University hosted by Prof. Michael
Mitzenmacher. He completed his Ph.D. in computer science from the
University of Maryland in 2019. He is interested in approximation
algorithms and algorithmic game theory. His research has been
supported by Ann. G. Wylie Fellowship, Algorithms Award, Larry Davis
Dissertation Award, and the University of Maryland's Dean's
Fellowship. Prior to joining Maryland, Saeed received his B.S. from
the Sharif University of Technology.
- July 8 (Friday), JST 15:55-16:20
Hitomi Yanaka (University of Tokyo)
"Exploring the Generalization Ability of Neural Models through
Natural Language Inference"
Abstract:
Modern deep neural networks have shown impressive performance in
various language understanding tasks, including semantically
challenging tasks such as Natural Language Inference (NLI). However,
recent analyses have pointed out that these neural models might learn
undesired biases or heuristics in the training data. It remains
unclear to what extent neural models have the generalization capacity
to perform arbitrary types of lexical and structural inferences. In
this invited talk, I introduce and discuss my recent work on
investigating whether neural models can learn the generalization
ability in natural language semantics. A series of experiments show
that current models systematically draw inferences on unseen
combinations of lexical and structural inference when the syntactic
structures of the sentences are similar between the training and test
sets. However, the performance of the models significantly decreases
when the structures are changed in the test set while retaining all
vocabularies and constituents already appearing in the training set.
This indicates that the generalization ability of neural models is
limited to cases where the syntactic structures are nearly the same as
those in the training set.
Bio:
Hitomi Yanaka received the Ph.D. degree in engineering from the
University of Tokyo, Japan, in 2018. From 2018 to 2021, she was a
postdoctoral researcher at the Riken Center for Advanced Intelligence
Project (AIP). She is currently a lecturer (Excellent Young
Researcher) at the Graduate School of Information Science and
Technology, the University of Tokyo. She is also a visiting researcher
at the Riken AIP and a part-time lecturer at Ochanomizu University.
She has also organized the International Workshop Natural Logic Meets
Machine Learning (NALOMA) from 2020. Her research interests include
computational linguistics and natural language processing, with focus
on Natural Language Inference.
- July 8 (Friday), JST 16:20-16:45
Shin-ichi Maeda (Preferred Networks)
"Meta-learning for generalization"
Abstract:
Machine learning has shown its remarkable ability for solving tasks in
various domains based on the given examples or experiences by
supervised learning or reinforcement learning. However, the trained
model becomes often weak or useless for the different tasks where the
task setting is different from the one in the training even if the
difference looks subtle. This problem has been treated as a type of
"overfitting", but in recent years, the researchers consider more from
the perspective how similar experiences can be used to learn new
tasks. It is sometimes called "transfer learning", but it is also
called "life-long learning" in the sense that all the past experience
is continuously utilized to quickly learn the new task, or "few-shot
learning" in the sense that a new task is learned with a small amount
of the task-specific data based on the previous experiences in the
learning of similar tasks. It is also called "meta-learning" to mean
the learning to learn. Here, I explain that this meta-learning problem
can be formulated as a variant of Bayes risk minimization problem, and
that the estimation of the posterior distribution becomes the key to
solve this problem. The estimation of the posterior distribution
requires a quick construction based on a small but variable size of
dataset given for each task. I also present a tractable Gaussian
approximation for this Bayesian posterior. Finally, I will introduce
some applications that use meta-learning: the estimation of the 3D
object shape from given small number of 3D points cloud and the policy
generalization that enables quick learning in novel environment which
has a novel dynamics parameter.
Bio:
Shin-ichi Maeda received the B.E. and the M.E. degrees in electrical
and engineering from Osaka University, and the Ph.D. degree in
information science from Nara Institute of Science and Technology,
Nara, Japan, in 2004. He is currently a senior researcher at Preferred
Networks, Inc. His current research interests are in machine learning,
in particular, Bayesian inference, reinforcement learning, and its
applications.
- July 8 (Friday), JST 16:45-17:10
Mayu Otani (Cyberagent)
"Evaluation of vision tasks: performance measures and datasets"
Abstract:
I will highlight issues in performance evaluation in computer vision
tasks. Many research fields rely on shared benchmarks that provide
datasets and evaluation pipelines to validate algorithms. The way we
evaluate algorithms harnesses the direction of the field; however,
most efforts are devoted to algorithm development, and there is less
effort to improve evaluation pipelines. This talk will introduce our
recent works on evaluating computer vision tasks, including video
summarization, natural language video localization, and object
detection.
Bio:
Mayu Otani obtained her Ph.D. at Nara Institute of Science and
Technology, Japan in 2018. She is currently a Research Scientist at
CyberAgent, Japan. Her work focuses on visual understanding,
especially analyzing evaluation measures and datasets in visual
understanding tasks.
- July 8 (Friday), JST 17:10-17:35
Ryo Yonetani (Omron SINIC X Corporation)
"Path Planning meets Machine Learning"
Abstract:
Path planning is a leading challenge in the field of AI, where many
excellent algorithms have been studied. In recent years, we have been
working on improving the performance of path planning algorithms with
modern machine learning techniques. In this talk, we will present our
latest achievements on improving the efficiency of classical A* search
and more advanced multi-agent path planning by utilizing deep
learning.
Bio:
Ryo Yonetani is a principal investigator at OMRON SINIC X and also a
project senior assistant professor at Keio University. He was
previously an assistant professor at University of Tokyo. He received
a PhD in Informatics from Kyoto University in 2013. His research
interests include machine learning (federated learning, transfer
learning, and neural planners) and computer vision (first person
vision and visual forecasting).
- July 9 (Saturday), JST 11:00-11:25
Komei Sugiura (Keio University)
"Semantic Machine Intelligence for Domestic Service Robots"
Abstract:
Increasing demand for support services for older and disabled people
has spurred the development of domestic service robots as an
alternative and credible solution to the shortage of caring
labor. Meanwhile, advances in vision and language technology have
brought about remarkable progress in grounded language processing for
embodied agents and physical robots. In this talk, I will present our
work on semantic machine intelligence, including sim2real, vision and
language navigation, visual referring expression comprehension, and
crossmodal language generation.
Bio:
Komei Sugiura is Professor at Keio University, Japan. He obtained a
B.E. in electrical and electronic engineering, and an M.S. and a
Ph.D. both in informatics from Kyoto University in 2002, 2004, and
2007, respectively. From 2006 to 2008, he was a research fellow at
Japan Society for the Promotion of Science. From 2006 to 2009, he was
also with ATR Spoken Language Communication Research
Laboratories. From 2008 to 2020, he was Senior Researcher at National
Institute of Information and Communications Technology, Japan, before
joining Keio University in 2020. His research interests include
multimodal language understanding, service robots, machine learning,
spoken dialogue systems, cloud robotics, imitation learning, and
recommender systems. He has won awards at international conferences
and competitions including RoboCup 2012, IROS 2018, and World Robot
Summit 2018.
- July 9 (Saturday), JST 11:25-11:50
Brian Bullins (Toyota Technological Institute at Chicago)
"Beyond First-Order Methods for Large-Scale Optimization"
Abstract:
In recent years, stochastic gradient descent (SGD) has taken center
stage for training large-scale models in machine learning. Although
methods which go beyond first-order information may achieve better
iteration complexity in theory, the per-iteration costs often render
them unusable when faced with the current growth in both the available
data and the size of the models, particularly when such models now
have hundreds of billions of parameters.
In this talk, I will present results, both theoretical and practical,
for dealing with a key challenge in this setting, whereby I will show
how second-order optimization may be as scalable as first-order
methods. Namely, optimization methods which may parallelize have also
become increasingly critical when facing enormous deep learning
models, and so I will show how we may leverage stochastic second-order
information to attain faster methods in the distributed optimization
setting.
Bio:
Brian Bullins is a research assistant professor at the Toyota
Technological Institute at Chicago. He received his Ph.D. in computer
science at Princeton University, where he was advised by Elad Hazan,
and his research was supported by a Siebel Scholarship. His interests
broadly lie in both the theory and practice of optimization for
machine learning. In particular, his work on improving matrix
estimation techniques has led to new second-order methods for convex
and nonconvex optimization with provable guarantees, along with
further applications for distributed settings, and his work has
received a best paper award at COLT 2021.
- July 9 (Saturday), JST 15:50-16:15
Yusuke Sekikawa (Denso IT laboratory)
"Event-based vision: detect and process sparse changes"
Abstract:
Recently, bio-inspired vision sensors called event-based cameras,
which capture brightness ``changes'', have received much attention
because of their advantages over conventional frame-based cameras.
One of the significant advantages is a sparse data representation that
allows high temporal resolution. A difference-driven neuron model
called ``Sigma-Delta'' is gaining much attention to exploit the
temporal sparsity. It is orders of magnitude more efficient than the
conventional dense neuron model because it processes only ``changes'',
and SoCs designed for the neuron model have emerged on the market. In
this talk, we'll share the basics of event-based cameras and discuss
the potential benefits of the difference-driven neuron model for
efficient spatiotemporally sparse data such as events or video
streams.
Bio:
Yusuke Sekiawa received his B.S. degree in electrical engineering from
the Science University of Tokyo, Japan, in 2004. From 2004 to 2009,
he worked at the Japanese Ministry of Economy Trade and Industry as a
patent examiner. From 2008 to 2012, he worked as a software engineer
at Olympus Imaging Co. Ltd. Since 2012, he joined DENSO IT Laboratory
at Shibuya, Tokyo, Japan as a computer vision researcher. From
2014-2015 he worked at the MIT Media Lab as a visiting scientist. In
2020, he received his Ph.D. degree in computer science at Keio
University. In 2020, he was cross-appointed to the Tokyo Institute of
Technology as a part-time teacher. His research interests include
machine learning and neuromorphic image processing.
- July 9 (Saturday), JST 16:15-16:40
Yasuhide Miura (Fuji Film Business Innovation)
"Neural Image-to-Text Models for Automatic Generation of Medical Report"
Abstract:
As a new application of natural language generation, researchers have
worked to build assistive systems that take medical images of a
patient and generate a textual report describing clinical observations
in the images. This kind of application is clinically important,
offering the potential to reduce medical workers' repetitive work and
generally improve clinical communication. In this talk, I will briefly
go over previous work that has tackled to generate medical reports
from radiology images and further describe our work that improved the
factual completeness and consistency of medical report generation.
Bio:
Yasuhide Miura is a research principal at FUJIFILM Business Innovation
Corp., Japan. His research interest is in applications of natural
language processing technologies especially to texts in medical
domain. He joined Fuji Xerox Co., Ltd. in 2004, has been a part-time
project researcher at the University of Tokyo Hospital from 2010 to
2012, has received D.Eng. from Tokyo Institute of Technology in 2019,
and has been a visiting scholar at Stanford University from 2019 to
2021.
- July 9 (Saturday), JST 16:40-17:05
Bradly Stadie (Toyota Technological Institute at Chicago)
"Making Sense of the Past with Hindsight Divergence Minimization"
Abstract:
How should intelligent agents ideally process and learn from past
experiences? Hindsight Experience Replay (HER) gives reinforcement
learning agents a powerful method for learning not only from past
successes, but also past failures as well. Yet, the HER family of
algorithms are very sensitive to the rewards an agent receives. This
problem is so pronounced an agent receiving (0, 1) rewards performs
much worse than an agent receiving (-1, 0) rewards, even though this
is a simple linear scaling of the reward function! We show,
concretely, why past Hindsight Experience Replay methods have been so
brittle. The trick, it turns out, lies in a fairly obscure bit of math
from information theory. Using this trick, we are able to finally
derive mathematically rigorous hindsight experience replay algorithms,
a long standing goal of reinforcement learning. By helping agents make
sense of their own past, we show our algorithms increase robotic
learning speed by an order of magnitude.
Bio:
Bradly Stadie is a Research Assistant Professor at the Toyota
Technological Institute at Chicago (TTIC). Before joining TTIC, he
received his PhD in Statistics from UC Berkeley in 2018. From
2016-2018, Bradly was also a research scientist at OpenAI, where he
was a founding member of the reinforcement learning team. Bradly's
current research centers on generalization in machine learning and
statistics, with an emphasis on test-time inference. This work draws
surprising connections to a variety of fields, including graphical
models, generative adversarial networks, causal inference, and graph
search. In September 2022, Bradly will start an appointment as an
Assistant Professor of Statistics at Northwestern University in
Chicago.
- July 9 (Saturday), JST 17:05-17:30
Makoto Miwa (Toyota Technological Institute)
"Information Extraction from Text with Heterogeneous Knowledgebase Information"
Abstract:
Information extraction from text aims to structure information in
unstructured text. In recent years, many neural extraction models have
been proposed. Most of them target only textual information, but
external information may be useful to understand the contents of such
texts. For example, drug entities in pharmacological texts are linked
to external drug databases, which may contain different types of
information such as entity descriptions, chemical structures, and
their related entities. Incorporating external heterogeneous
information can enhance extraction in a variety of ways, including
building training data and enriching information about the entities
and relationships to be extracted. This talk will introduce our recent
efforts to incorporate and utilize such external information.
Bio:
Makoto Miwa is an associate professor at Toyota Technological
Institute (TTI). He is also an invited senior researcher at the
Artificial Intelligent Research Center (AIRC), National Institute of
Advanced Industrial Science and Technology (AIST). He received his
Ph.D. from the University of Tokyo in 2008. His research mainly
focuses on information extraction from texts, deep learning, and
representation learning.
|